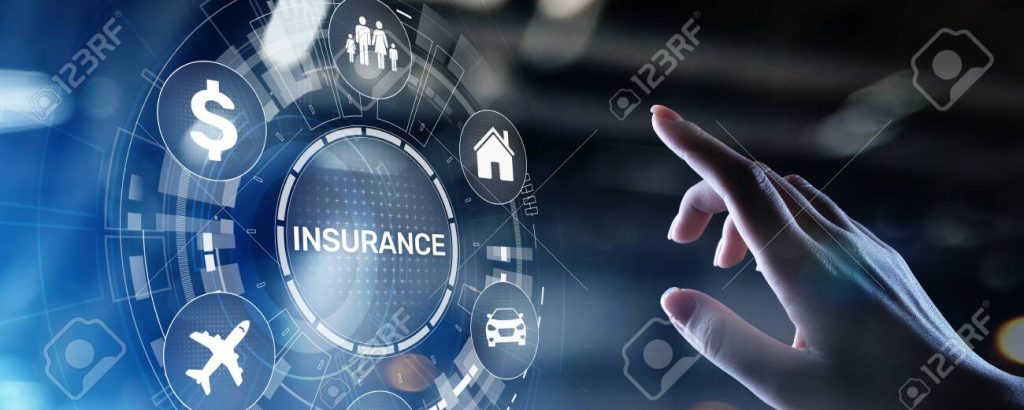
The insurance industry is regarded as one of the most competitive and less predictable business spheres. It is instantly related to risk. Therefore, it has always been dependent on statistics. Nowadays, data science has changed this dependence forever.
Now, insurance companies have a wider range of information sources for the relevant risk assessment. Big Data technologies are applied to predict risks and claims, to monitor and to analyse them in order to develop effective strategies for customers attraction and retention. Undoubtedly, the insurance companies benefit from data science application within the spheres of their great interest. Therefore, we have prepared the top 10 data science use cases in the insurance industry, which cover many various activities.
Fraud detection
Insurance fraud brings vast financial loss to insurance companies every year. Data science platforms and software made it possible to detect fraudulent activity, suspicious links, and subtle behavior patterns using multiple techniques.
To make this detection possible the algorithm should be fed with a constant flow of data. Usually, insurance companies use statistical models for efficient fraud detection. These models rely on the previous cases of fraudulent activity and apply sampling method to analyze them. In addition, predictive modeling techniques are applied here, for the analysis and filtering of fraud instances. Identifying links between suspicious activities helps to recognize fraud schemes that were not noticed before.
Price optimization
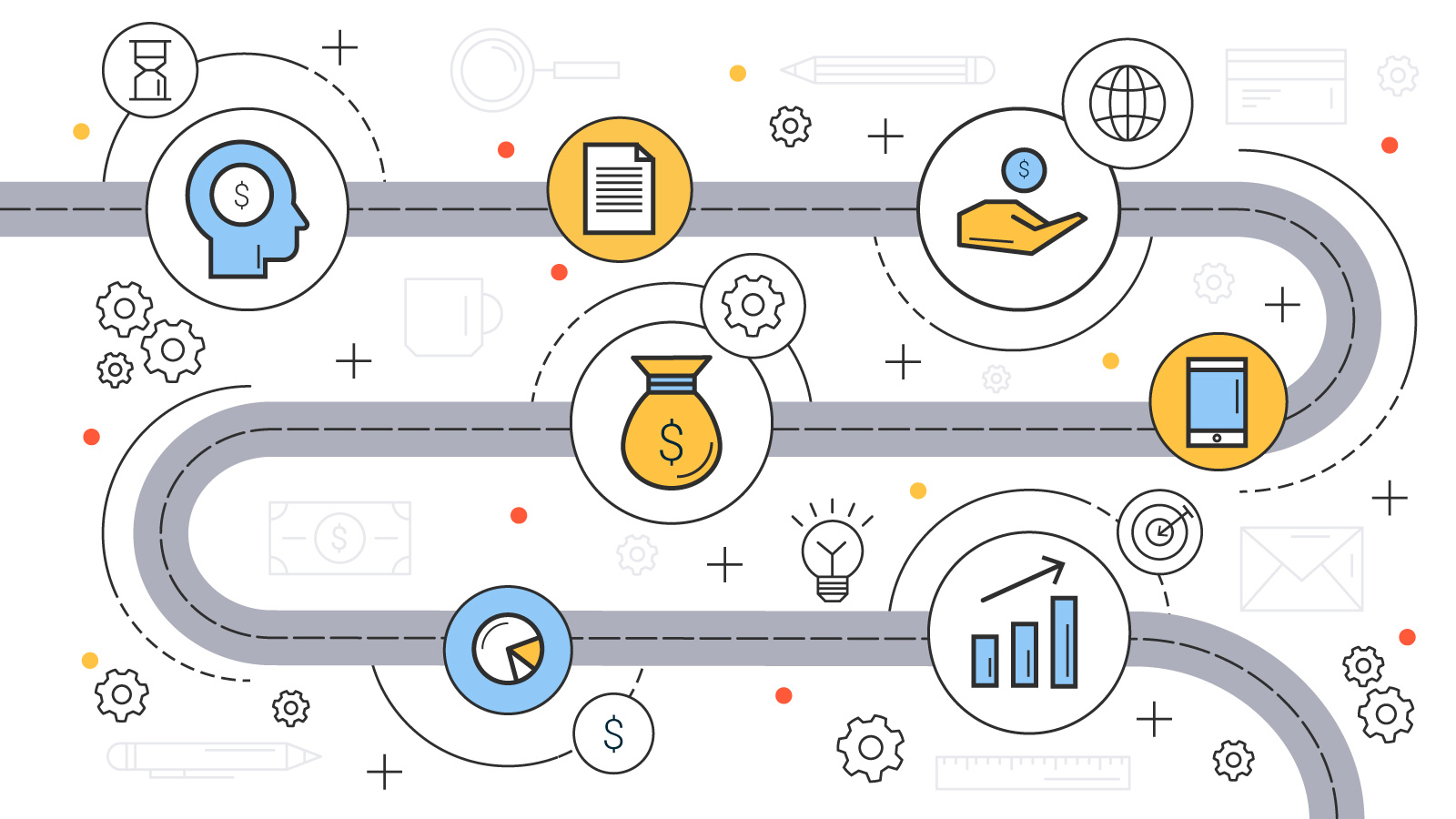
Price optimization procedure is a complex notion. Therefore it uses numerous combinations of various methods and algorithms. Despite the fact that it is still the disputable issue of applying this procedure for insurance, more and more insurance companies adopt this practice.
This process supposes combining the data not related to the expected costs and risk characteristics and the data not related to the expected loss and expenses, and its further analysis. That is, it takes into consideration the changes in comparison to the previous year and policy. Thus, price optimization is closely related to the customers’ price sensitivity.
In other words, historical costs, expenses, claims, risk, and profit are projected into the future. Special algorithms give the insurers the opportunity to adjust the quoted premiums dynamically.
As a key positive feature, price optimization helps to increase the customers’ loyalty in long perspective. Along with this, comes the maximization of profit and income.